
In today’s day and age, the biggest asset for businesses is data. The more data they have access to, the more insights they can generate. Through data, they can see patterns that no one knew existed. This further helps them to make more informed decisions and stay ahead of the curve. Data science is basically a multidisciplinary field that essentially focuses on extracting insights from large data sets – both raw and structured.
What Is Data Analytics?
By defining data analytics we’ll come a little closer to understanding data science vs data analytics. Data analytics comes under the purview of data science. It essentially processes and performs statistical analysis on the existing sets of data. So data analytics is not about finding questions but finding answers and gaining insights for problems that we know.
Unlike data science, here we already have a set of questions around which we are supposed to work. Data analytics, though related to data science, is much limited in its scope and is much more specific. It does not aim to look for connections between the data but ways to support the goal in mind. Precisely, data analytics analyzes raw data to make conclusions about that information. The techniques of analytics are used in organizations for making better and informed decisions and by scientists for verifying or disproving theories and scientific models.
What is Artificial Intelligence?
Artificial intelligence is nothing but the simulation of human intelligence in machines. AI enables machines to think, learn, and find solutions (solve problems) just like human brains do. AI possesses the power to rationalize like us and take actions that are most likely to accomplish a goal. Through artificial intelligence, machines can execute the desired tasks by imitating human intelligence.
What is Machine Learning?
Machine learning is a subset of artificial intelligence. It essentially gives machines the ability to learn and improve through experiences – without the need to program them explicitly. ML aims to develop programs that can access data and utilise it to learn for themselves.
Data Science vs Data Analytics vs Machine Learning vs Artificial Intelligence- Careers
Aspects | Data Science | Data Analytics | Machine Learning | Artificial Intelligence |
Job roles | Data Engineer, Data Scientist, Data Analyst, Data Architect,Database Administrator, Machine Learning Engineer, Statistician,Business Analyst, Data and Analytics Manager. | Sales Analyst, Operations Analyst, Customer Success Analyst, Market Research Analyst, Marketing Analyst, Business Analyst, Financial Analyst, and more. | Machine Learning Engineer, Data Architect, Data Scientist, Data Mining Specialist, Cloud Architect, and Cyber Security Analyst, and more. | Machine Learning Engineer, Data Scientist,Business Intelligence Developer,Big Data Architect, Research Scientist. |
Skills | Programming Skills.Statistics.Machine Learning. Multivariable Calculus & Linear AlgebraData Visualization & CommunicationSoftware Engineering.Data Intuition. | Mathematical skills, Programming languages- SQL, Oracle and Python.Ability to analyse, model and interpret data. Problem-solving skills. | StatisticsProbabilityData ModelingPrograming SkillsApplying ML Libraries & Algorithms, Software Design, Python | Mathematical and Algorithms skills, Probability and Statistics knowledge, Expertise In Programming – Python, C++, R, JavaWell-versed with Unix Tools, Awareness about Advanced Signal Processing Techniques. |
Artificial Intelligence vs Machine Learning
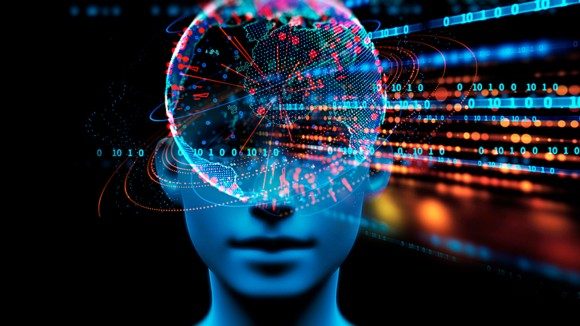
- Artificial intelligence essentially makes machines simulate human intelligence while ML deals with learning from past data without being explicitly programmed.
- AI focuses on making systems that can solve complex problems while ML aims to make machines learn from available data and generate accurate outputs.
- Subsets of AI – machine learning and deep learning while a subset of machine learning – deep learning.
- AI works towards maximizing the chances of success while ML is concerned with understanding patterns and giving accurate results.
- AI involves the process of learning, reasoning, and self-correction while ML deals with learning and self-correction only when introduced to new data.
- Artificial Intelligence deals with structured, unstructured, and semi-structured data while Machine learning deals only with structured and semi-structured data.
Data Science vs Artificial Intelligence
- Data science deals with pre-processing, analysing, visualizing, and predicting the data. Whereas, AI implements a predictive model used for forecasting future events.
- Data science banks on statistical techniques while AI leverages computer algorithms.
- The tools used in data science are much more in quantity than the ones used in AI. The reason for this is – there are multiple steps for analyzing data and extracting insights from it.
- In data science, the focus remains on building models that use statistical insights, whereas, for AI, the aim is to build models that can emulate human intelligence.
- Data science strives to find hidden patterns in the raw and unstructured data while AI is about assigning autonomy to data models.
Data Analytics vs Machine Learning
- Analytics relies on existing information to find patterns that ultimately shape decisions. Whereas machine learning leverages existing data that provides the base for the machine to learn for itself.
- Analytics reveals patterns through the process of classification and analysis while ML uses the algorithms to do the same as analytics but in addition, learns from the collected data.
- Data analytics ultimately aims to find patterns whereas ML aims to learn from data and make estimates and predictions.

What Does an AI Engineer Do?
An AI engineer builds AI models using machine learning algorithms and deep learning neural networks to draw business insights, which can be used to make business decisions that affect the entire organization. These engineers also create weak or strong AIs, depending on what goals they want to achieve.
AI engineers have a sound understanding of programming, software engineering, and data science. They use different tools and techniques so they can process data, as well as develop and maintain AI systems.
The next section of How to become an AI Engineer focuses on the responsibilities of an AI engineer.
Responsibilities of an AI Engineer
As an AI engineer, you need to perform certain tasks, such as develop, test, and deploy AI models through programming algorithms like random forest, logistic regression, linear regression, and so on.
Responsibilities include:
- Convert the machine learning models into application program interfaces (APIs) so that other applications can use it
- Build AI models from scratch and help the different components of the organization (such as product managers and stakeholders) understand what results they gain from the model
- Build data ingestion and data transformation infrastructure
- Automate infrastructure that the data science team uses
- Perform statistical analysis and tune the results so that the organization can make better-informed decisions
- Set up and manage AI development and product infrastructure
- Be a good team player, as coordinating with others is a must
Skills Required to Become an AI Engineer
Professionals who are finding how to become an AI engineer should also know about the skills required in this field. Some of them include:
Technical Skills
- Programming Skills
The first skill required to become an AI engineer is programming. To become well-versed in AI, it’s crucial to learn programming languages, such as Python, R, Java, and C++ to build and implement models.
- Linear Algebra, Probability, and Statistics
To understand and implement different AI models—such as Hidden Markov models, Naive Bayes, Gaussian mixture models, and linear discriminant analysis—you must have detailed knowledge of linear algebra, probability, and statistics.
- Spark and Big Data Technologies
AI engineers work with large volumes of data, which could be streaming or real-time production-level data in terabytes or petabytes. For such data, these engineers need to know about Spark and other big data technologies to make sense of it. Along with Apache Spark, one can also use other big data technologies, such as Hadoop, Cassandra, and MongoDB.
- Algorithms and Frameworks
Understanding how machine learning algorithms like linear regression, KNN, Naive Bayes, Support Vector Machine, and others work will help you implement machine learning models with ease. Additionally, to build AI models with unstructured data, you should understand deep learning algorithms (like a convolutional neural network, recurrent neural network, and generative adversarial network) and implement them using a framework. Some of the frameworks used in artificial intelligence are PyTorch, Theano, TensorFlow, and Caffe.
Non-Technical Skills
- Communication and Problem-Solving Skills
AI engineers need to communicate correctly to pitch their products and ideas to stakeholders. They should also have excellent problem-solving skills to resolve obstacles for decision making and drawing helpful business insights.
Let us explore the career and roles in AI in the next section of the How to become an AI Engineer article.
Few ways / roles to Build a Career in AI
Since several industries around the world use AI to some degree or the other, including healthcare and education, there has been exponential growth in the career opportunities within the field of AI. Some of these job roles are:
AI Developer
An AI developer works closely with electrical engineers and develops software to create artificially intelligent robots.
AI Architect
AI architects work closely with clients to provide constructive business and system integration services. They also create and maintain the entire architecture.
Machine Learning Engineer
Machine learning engineers build predictive models using vast volumes of data. They have in-depth knowledge of machine learning algorithms, deep learning algorithms, and deep learning frameworks.
Data Scientists
Data scientists collect, clean, analyze, and interpret large and complex datasets by leveraging both machine learning and predictive analytics.
Business Intelligence Developer
They’re responsible for designing, modeling, and analyzing complex data to identify the business and market trends.